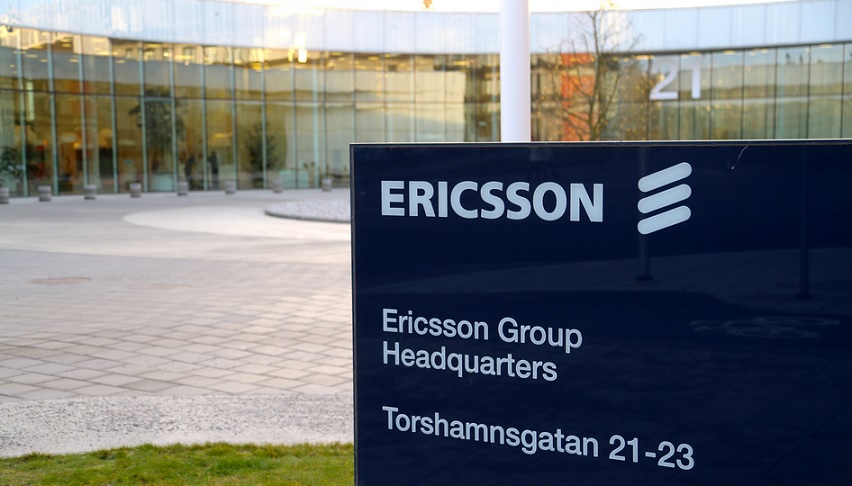
Researchers at Ericsson have offered a unique, ‘Tiny-ML-as-a-service‘ concept to address some of the challenges faced in machine learning (ML) in the Internet of Things (IoT) today.
In a new post as part of an on-going series on the company official blog, the team has pointed out that despite the use of ML, many technological barriers make it “challenging” to realise the full value of ML-driven IoT at the Edge. It has proposed the idea of a tiny machine learning as a solution, laying out the possibilities and challenges of ML for IoT, and introducing the concept of TinyML as-a-service.
The Ericsson research team has highlighted the fact that there was no formal and uniform view on what the de facto ML in IoT meant. This had led to multiple opportunities and interpretations.
In our view, taking advantage of intelligent algorithms in the IoT context translates to also having the possibility to equip IoT end-devices (such as sensors, actuators and micro-controllers) with functionalities capable of unleashing the power of ML algorithms on the IoT device itself. This thus extends the use of ML in IoT beyond in the cloud and more capable devices running e.g. Linux.
In most cases, many consider ML in IoT as having the possibility to provide ML inference capabilities to devices similar to the well-known Single-Board Computer “Raspberry Pi”, with the latter taking the role of IoT device. The question is then, how can we put ML algorithms on constrained devices that are 32-bit MCUs and not capable of running an OS like Linux.
– Ericsson
Detailing the “explicit definition of a constrained IoT device” as a start-off point, the research team explained it was crucial to characterise the distinction between “serving” machine learning to IoT devices, and “developing” machine learning within IoT devices.
In the first case, all the ML-related tasks are “outsourced” to the Edge and Cloud, meaning that an IoT device is somehow “passively” waiting to receive the rendered ML model algorithm elaboration.
In the second case, conversely, an IoT device concretely takes part in the execution of intelligent services. This is defined as TinyML as-a-Service, regardless of the more limited resources compared to Cloud and Edge.
The team also laid out the challenges in this form of a service:
- the technical challenges within Edge computing and how it cannot be applied to solve all problems
- the relevance of technological differences between Web-based and embedded worlds, especially from deployment and execution perspectives
- the demand which ML places on computational resources for delivering an accurate and reliable experience
Ericsson will be putting out a whole lot more on this subject to further explain how the TinyML as-a-Service approach “can bridge the worlds of constrained IoT and ML together. “
Image Credit: Ericsson